ASSIST FEATURES
Sub-resolution assist features (SRAFs) are inserted in mask layout to improve the manufacturing process window of main patterns. SRAFs should be large enough to maximize their effect, but they are not intended to be printed on photoresist (PR). An accurate method of SRAF printing prediction is important to assure that no SRAFs are actually printed.
SRAF has evolved from a simple shape of narrow line to more complex two-dimensional curvilinear shape. Some SRAF printability check methods using machine learning model are also proposed. Inputs like Polar Fourier transform (PFT) signals and local layout densities are extracted from a pixel and its surroundings, and can be provided to MLP.
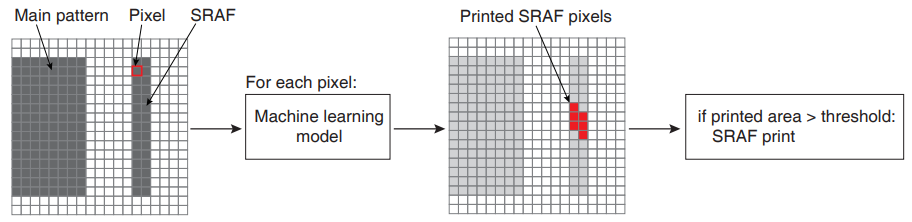
OPTICAL PROXIMITY CORRECTION
Optical proximity correction (OPC) is a lithography resolution enhancement technique and modifies a mask pattern to compensate for image errors due to light inference. Each edge of a mask pattern is divided into several segments. Each segment is individually shifted by a certain amount called mask bias. Lithography simulation is then used to obtain a photoresist contour. By comparing with a target pattern for each segment, edge placement error (EPE) values are calculated. Our lab is researching OPC using ANN or CNN models in order to predict fast and accurate OPC methods.
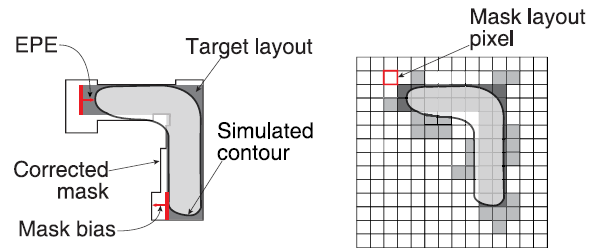
HOTSPOT
Lithography hotspots are the patterns that may cause defect such as bridging (short-circuit), necking (open-circuit), line-end shortening, etc. Hotspot detection is performed through pattern matching between layout patterns and hotspot patterns in a library. Layout regions with potential hotspots may be submitted to lithography simulations to confirm actual hotspots. Our goal is to generate reasonable hotspot patterns and predict hotspots before manufacturing.
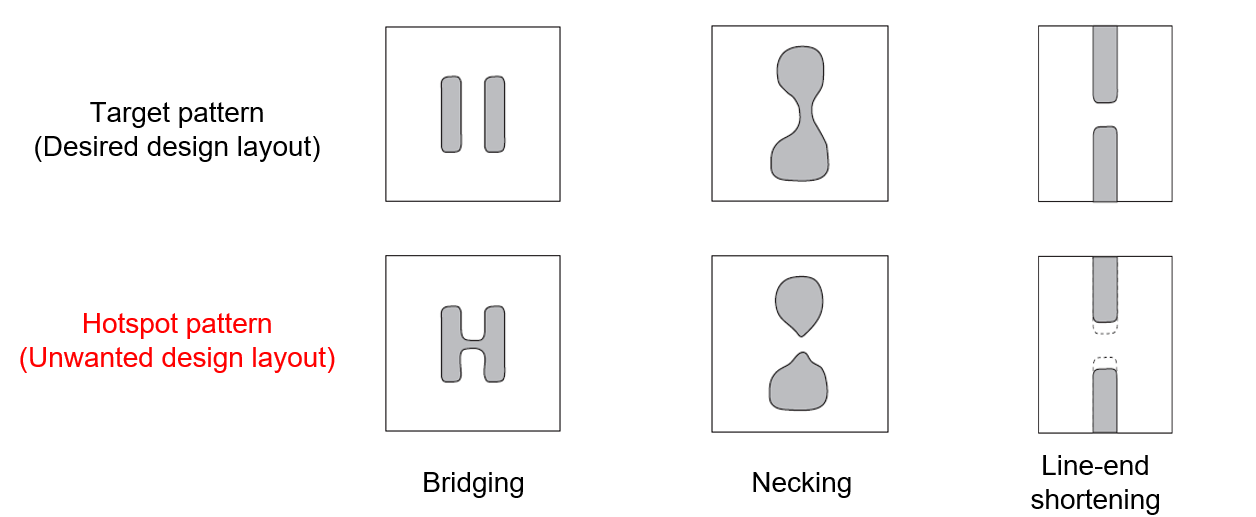
COMPACT SIMULATION
Model-based lithography simulation is used for fast simulation of 2-dimensional resist contour through two compact models: optical model, which corresponds to exposure step and yields an optical intensity map, and resist model, which is for development step and outputs the final resist contour.
Many kernel convolution contains in both optical model and resist model. To effectively accelerate the entire process, a more compact simulation way is needed. We aim to achieve a reduction in the number of kernels while maintaining or improving accuracy by using machine learning models or mathematical derivations.
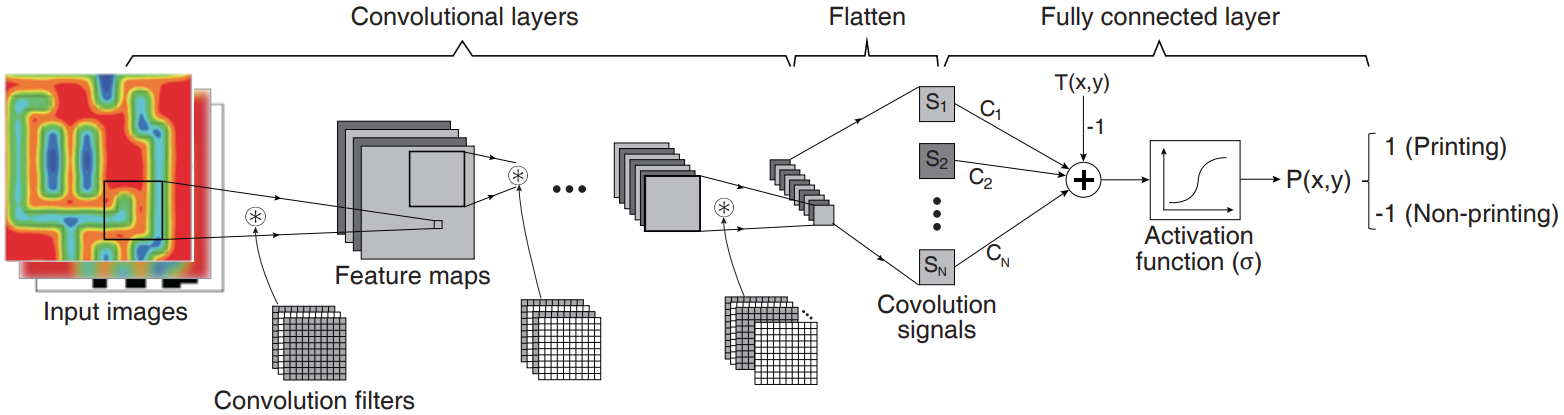