Computational Lithography
The lithography process is a process of exposing photoresist on a wafer by transmitting light through a mask in the shape of a design layout. As the miniaturization of the semiconductor process continues, the pattern of the mask also becomes small, and light passing through a narrow gap forms a photoresist with a distorted shape due to diffraction and interference.
Computational lithography is the process of correcting and verifying a mask image using a computer to accurately engrave a design layout on a wafer. The correction process includes optical proximity correction (OPC), sub-resolution assist feature (SRAF) insertion, etch proximity correction (EPC), and the verification process includes lithography simulation, resist profile simulation, and the like.
KEYWORDS OPC, EPC, SRAF
Related Publications SRAF printing prediction using artificial neural network
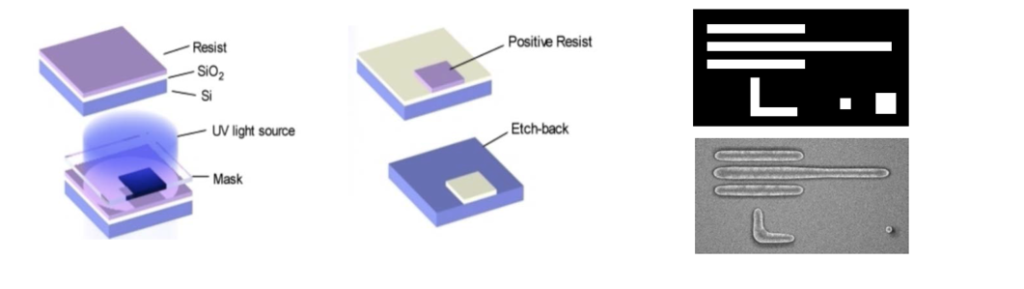
Machine Learning-Guided OPC (ML-OPC)
As the pattern of design layout becomes more refined, more accurate lithography simulation and mask image modification are required, and the time required for MB-OPC(model based OPC) is rapidly increasing.
ML-OPC repetitive lithography simulation bypassing , using a machine learning algorithm from the target layout OPC is a way to get the masked image directly. A design layout segment a parameter (eg pattern densities, optical signals kernel) and expressed as a neural network if the input , the segment of the mask bias is output. After obtaining mask bias for all segments, OPC mask image is drawn.
KEYWORDS OPC, NN
Related Publications Machine learning (ML)-guided OPC using basis functions of polar Fourier transform
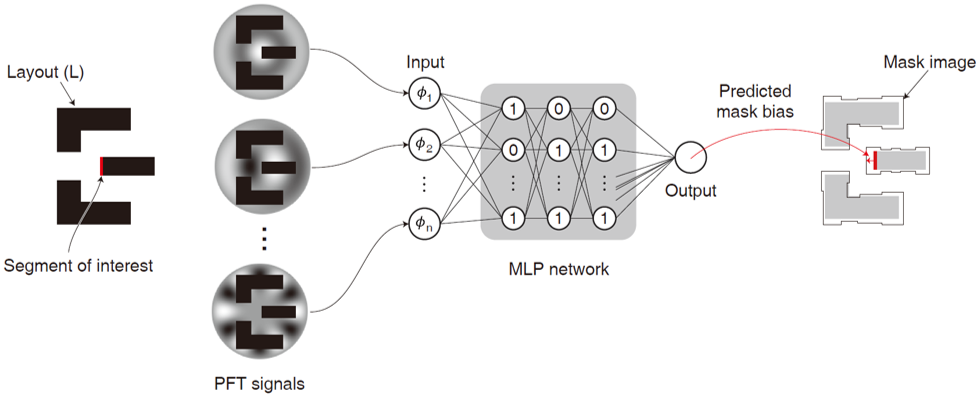
Layout Pattern Synthesis
Comprehensive test patterns of various shapes are important for many resource graphics applications. However, even if patterns are carefully extracted and classified from parametric patterns or real patterns, it is not easy to satisfy the diversity of patterns. We map the extracted layout pattern on the feature space to calibrate the lithographic model.
Calibration of the model with extracted patterns makes it well-predictable for patterns belonging to regions covered with learning data, but it is difficult to predict for layout patterns in empty areas on feature spaces. Therefore, this work aims to automatically generate new layouts to fill in empty areas on feature spaces. After generating the pattern, the model is calibrated using the synthesized layout with the existing layout. Adding a synthesized layout reduces the empty area on the feature space, allowing for more active acceptance of new patterns.
KEYWORDS Layout pattern, GAN
Related Publications Synthesis of lithography test patterns using machine learning model
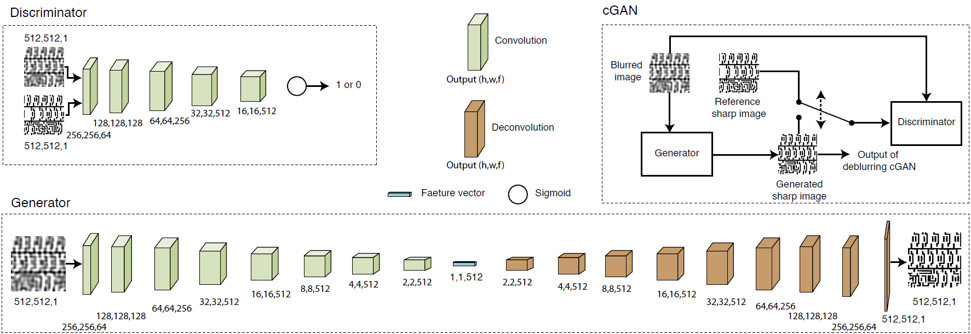